Visual Cue Effects on a Classification Accuracy Estimation Task in Immersive Scatterplots
Fumeng Yang, James Tompkin, Lane Harrison, David H. Laidlaw
DOI: 10.1109/TVCG.2022.3192364
Room: 106
2023-10-25T03:12:00ZGMT-0600Change your timezone on the schedule page
2023-10-25T03:12:00Z
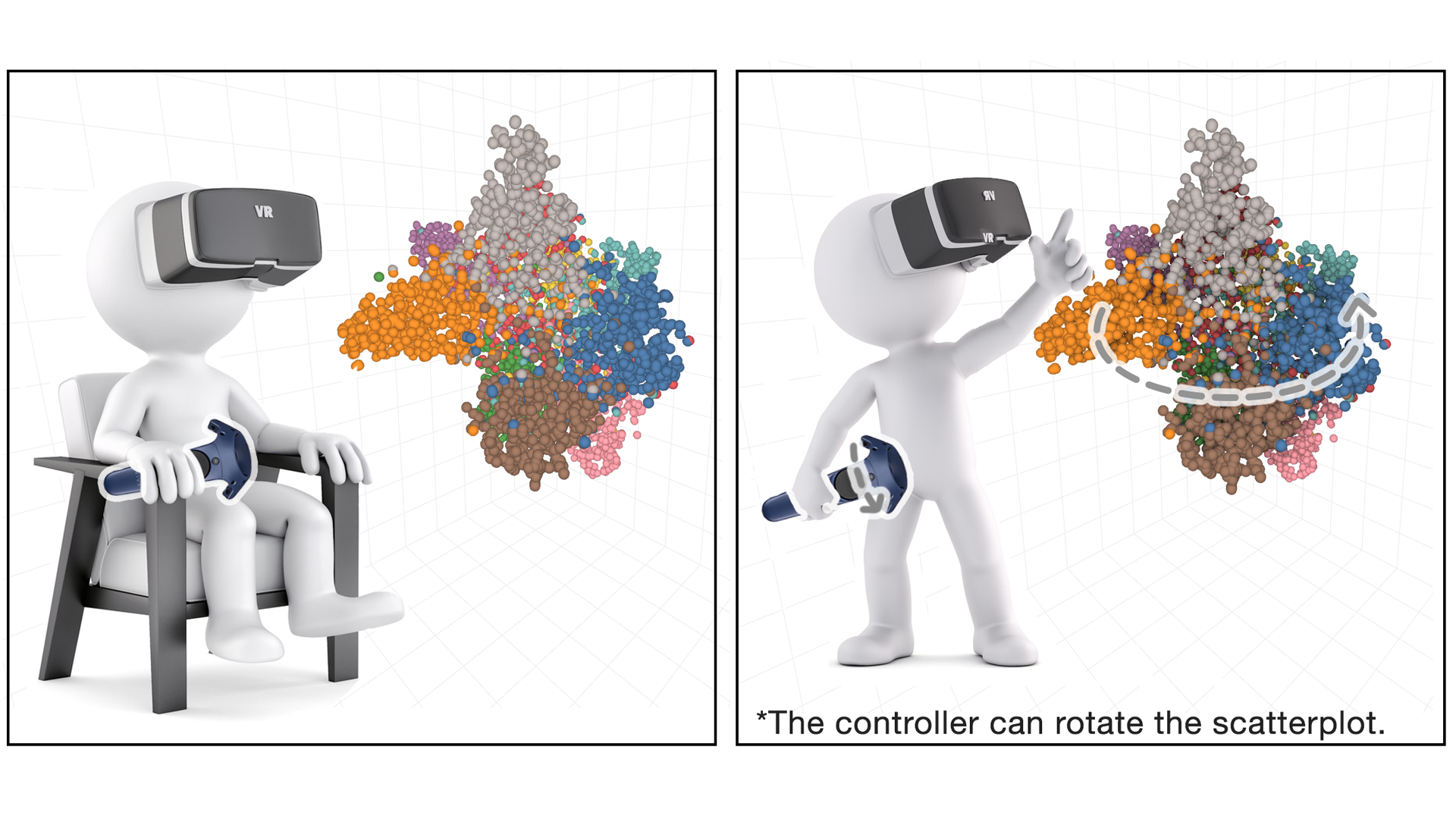
Fast forward
Full Video
Keywords
Virtual reality;cluster perception;information visualization;immersive analytics;dimension reduction;classification
Abstract
Immersive visualization in virtual reality (VR) allows us to exploit visual cues for perception in 3D space, yet few existing studies have measured the effects of visual cues. Across a desktop monitor and a head-mounted display (HMD), we assessed scatterplot designs which vary their use of visual cues—motion, shading, perspective (graphical projection), and dimensionality—on two sets of data. We conducted a user study with a summary task in which 32 participants estimated the classification accuracy of an artificial neural network from the scatterplots. With Bayesian multilevel modeling, we capture the intricate visual effects and find that no cue alone explains all the variance in estimation error. Visual motion cues generally reduce participants’ estimation error; besides this motion, using other cues may increase participants’ estimation error. Using an HMD, adding visual motion cues, providing a third data dimension, or showing a more complicated dataset leads to longer response times. We speculate that most visual cues may not strongly affect perception in immersive analytics unless they change people’s mental model about data. In summary, by studying participants as they interpret the output from a complicated machine learning model, we advance our understanding of how to use the visual cues in immersive analytics.