Toward Systematic Considerations of Missingness in Visual Analytics
Maoyuan Sun, Yue Ma, Yuanxin Wang, Tianyi Li, Jian Zhao, Yujun Liu, Ping-Shou Zhong
View presentation:2022-10-20T14:45:00ZGMT-0600Change your timezone on the schedule page
2022-10-20T14:45:00Z
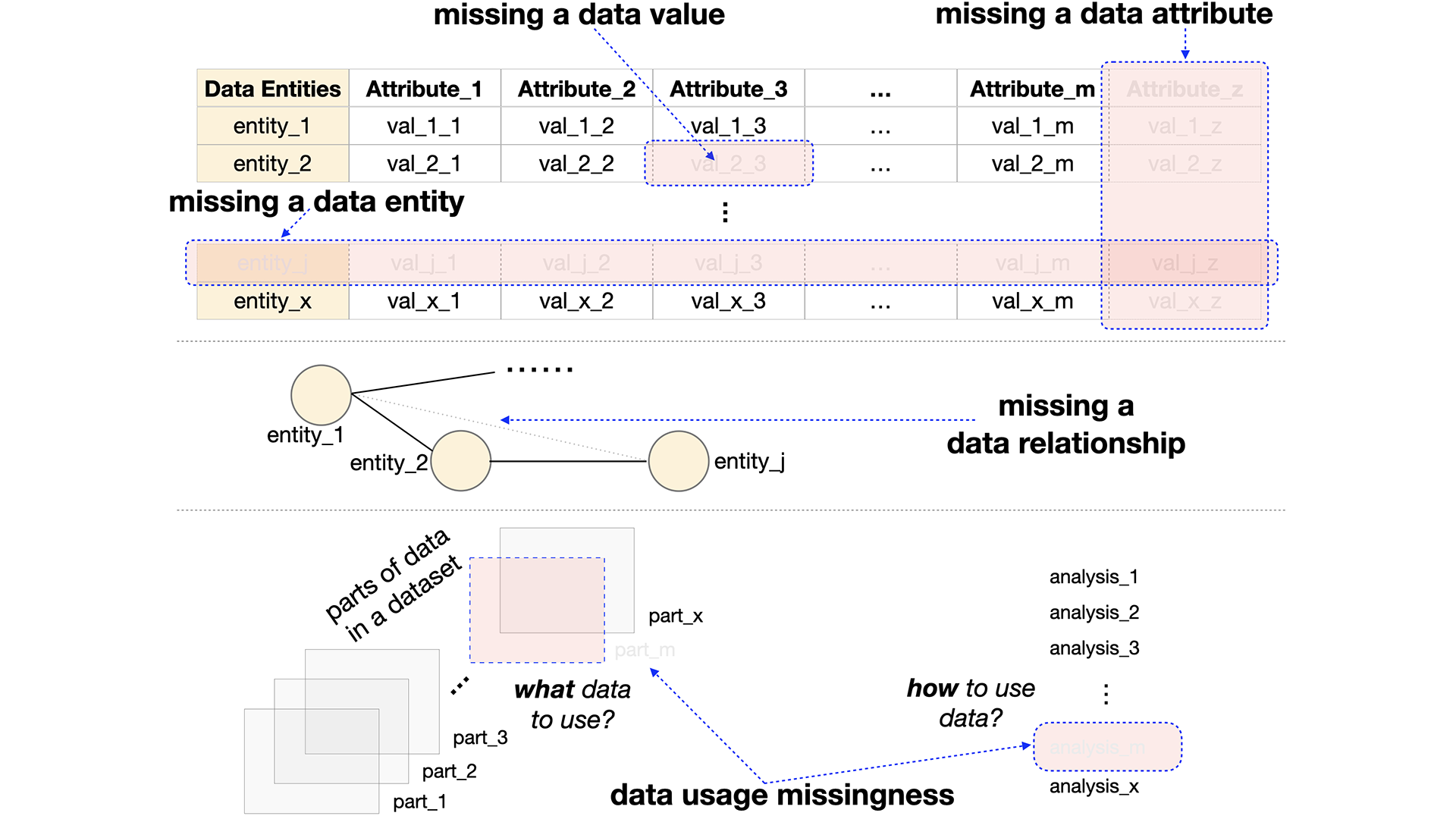
Prerecorded Talk
The live footage of the talk, including the Q&A, can be viewed on the session page, Personal Visualization, Theory, Evaluation, and eXtended Reality.
Fast forward
Keywords
Missingness, missing data visualization, sensemaking, visual analytics
Abstract
Data-driven decision making has been a common task in today’s big data era, from simple choices such as finding a fast way to drive home, to complex decisions on medical treatment. It is often supported by visual analytics. For various reasons (e.g., system failure, interrupted network, intentional information hiding, or bias), visual analytics for sensemaking of data involves missingness (e.g., data loss and incomplete analysis), which impacts human decisions. For example, missing data can cost a business millions of dollars, and failing to recognize key evidence can put an innocent person in jail. Being aware of missingness is critical to avoid such catastrophes. To fulfill this, as an initial step, we consider missingness in visual analytics from two aspects: data-centric and human-centric. The former emphasizes missingness in three data-related categories: data composition, data relationship, and data usage. The latter focuses on the human-perceived missingness at three levels: observed-level, inferred-level, and ignored-level. Based on them, we discuss possible roles of visualizations for handling missingness, and conclude our discussion with future research opportunities.