DPVisCreator: Incorporating Pattern Constraints to Privacy-preserving Visualizations via Differential Privacy
Jiehui Zhou, Xumeng Wang, Jason Kamkwai Wong, Huanliang Wang, Zhongwei Wang, Xiaoyu Yang, Xiaoran Yan, Haozhe Feng, Huamin Qu, Haochao Ying, Wei Chen
View presentation:2022-10-20T16:45:00ZGMT-0600Change your timezone on the schedule page
2022-10-20T16:45:00Z
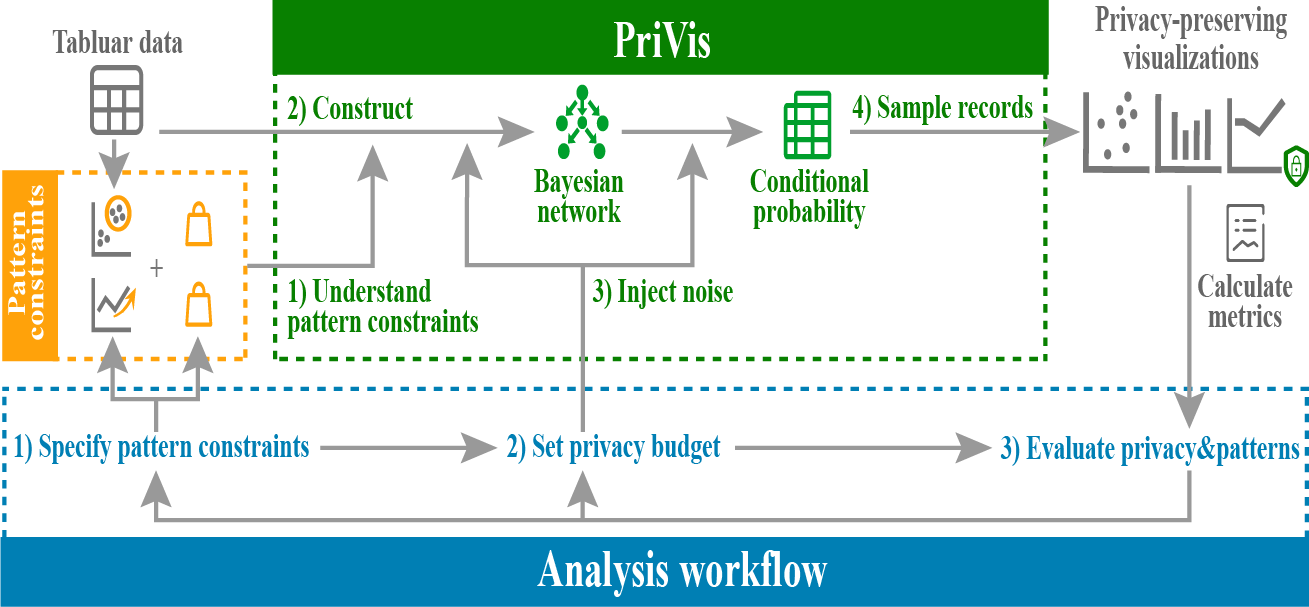
Prerecorded Talk
The live footage of the talk, including the Q&A, can be viewed on the session page, Digital Humanities, e-Commerce, and Engineering.
Fast forward
Abstract
Data privacy is an essential issue in publishing data visualizations. However, it is challenging to represent multiple data patterns in privacy-preserving visualizations. The prior approaches target specific chart types or perform an anonymization model uniformly without considering the importance of data patterns in visualizations. In this paper, we propose a visual analytics approach that facilitates data custodians to generate multiple private charts while maintaining user-preferred patterns. To this end, we introduce pattern constraints to model users' preferences over data patterns in the dataset and incorporate them into the proposed Bayesian network-based Differential Privacy (DP) model PriVis. A prototype system, DPVisCreator, is developed to assist data custodians in implementing our approach. The effectiveness of our approach is demonstrated with quantitative evaluation of pattern utility under the different levels of privacy protection, case studies, and semi-structured expert interviews.