Predicting User Preferences of Dimensionality Reduction Embedding Quality
Cristina Morariu, Adrien Bibal, Rene Cutura, Benoit Frenay, Michael Sedlmair
View presentation:2022-10-20T14:48:00ZGMT-0600Change your timezone on the schedule page
2022-10-20T14:48:00Z
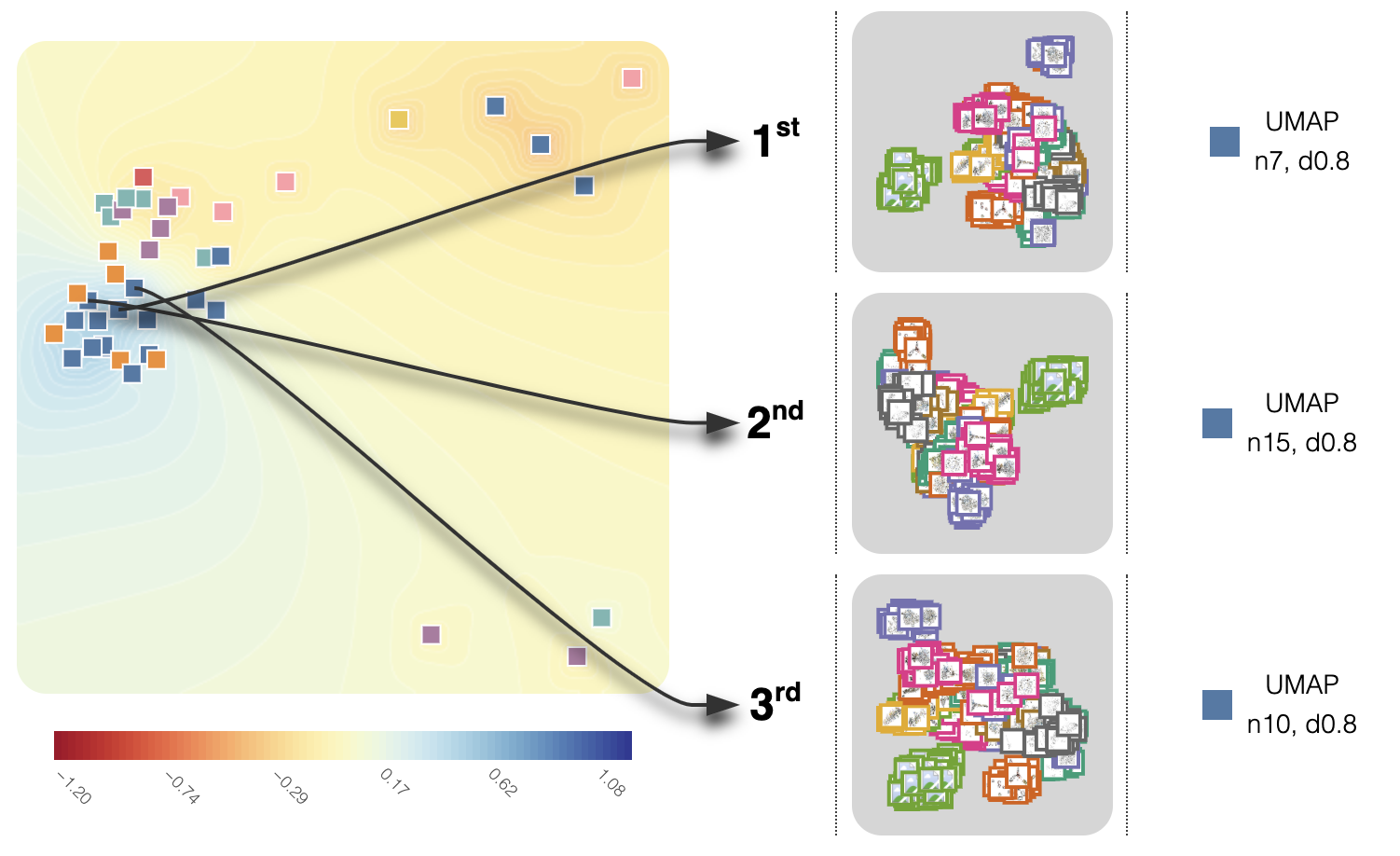
Prerecorded Talk
The live footage of the talk, including the Q&A, can be viewed on the session page, Interactive Dimensionality (High Dimensional Data).
Fast forward
Abstract
A plethora of dimensionality reduction techniques have emerged over the past decades, leaving researchers and analysts with a wide variety of choices for reducing their data, all the more so given some techniques come with additional hyper-parametrization (e.g., t-SNE, UMAP, etc.). Recent studies are showing that people often use dimensionality reduction as a black-box regardless of the specific properties the method itself preserves. Hence, evaluating and comparing 2D embeddings is usually qualitatively decided, by setting embeddings side-by-side and letting human judgment decide which embedding is the best. In this work, we propose a quantitative way of evaluating embeddings, that nonetheless places human perception at the center. We run a comparative study, where we ask people to select "good'' and "misleading'' views between scatterplots of low-dimensional embeddings of image datasets, simulating the way people usually select embeddings. We use the study data as labels for a set of quality metrics for a supervised machine learning model whose purpose is to discover and quantify what exactly people are looking for when deciding between embeddings. With the model as a proxy for human judgments, we use it to rank embeddings on new datasets, explain why they are relevant, and quantify the degree of subjectivity when people select preferred embeddings.