Incorporation of Human Knowledge into Data Embeddings to Improve Pattern Significance and Interpretability
Jie Li, Chunqi Zhou
View presentation:2022-10-20T14:24:00ZGMT-0600Change your timezone on the schedule page
2022-10-20T14:24:00Z
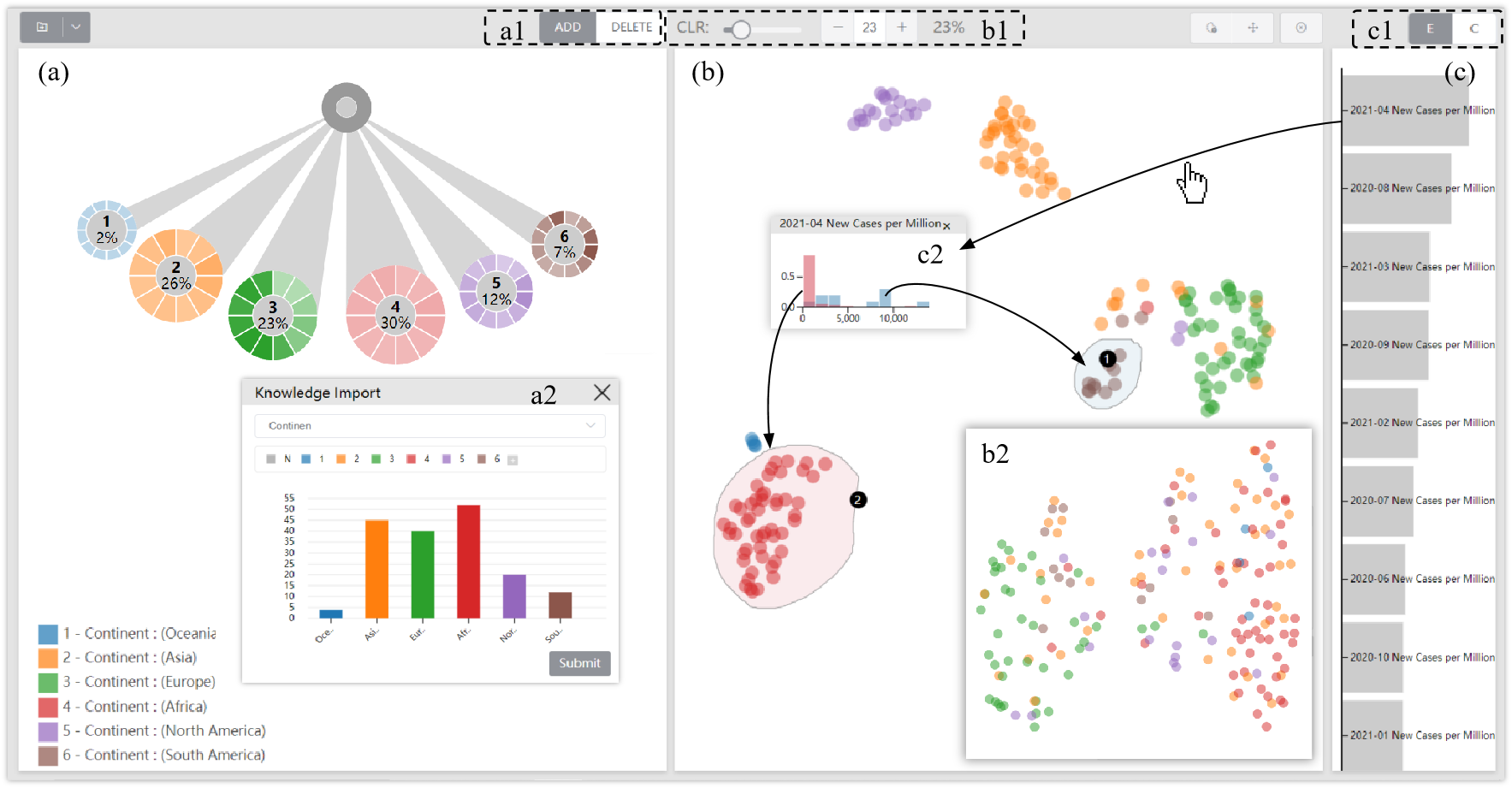
Prerecorded Talk
The live footage of the talk, including the Q&A, can be viewed on the session page, Interactive Dimensionality (High Dimensional Data).
Fast forward
Abstract
Embedding is a common technique for analyzing multi-dimensional data. However, the embedding projection cannot always form significant and interpretable visual structures that foreshadow underlying data patterns. We propose an approach that incorporates human knowledge into data embeddings to improve pattern significance and interpretability. The core idea is (1) externalizing tacit human knowledge as explicit sample labels and (2) adding a classification loss in the embedding network to encode samples’ classes. The approach pulls samples of the same class with similar data features closer in the projection, leading to more compact (significant) and class-consistent (interpretable) visual structures. We give an embedding network with a customized classification loss to implement the idea and integrate the network into a visualization system to form a workflow that supports flexible class creation and pattern exploration. Patterns found on open datasets in case studies, subjects’ performance in a user study, and quantitative experiment results illustrate the general usability and effectiveness of the approach.