Data Hunches: Incorporating Personal Knowledge into Visualizations
Haihan Lin, Derya Akbaba, Miriah Meyer, Alexander Lex
View presentation:2022-10-19T20:00:00ZGMT-0600Change your timezone on the schedule page
2022-10-19T20:00:00Z
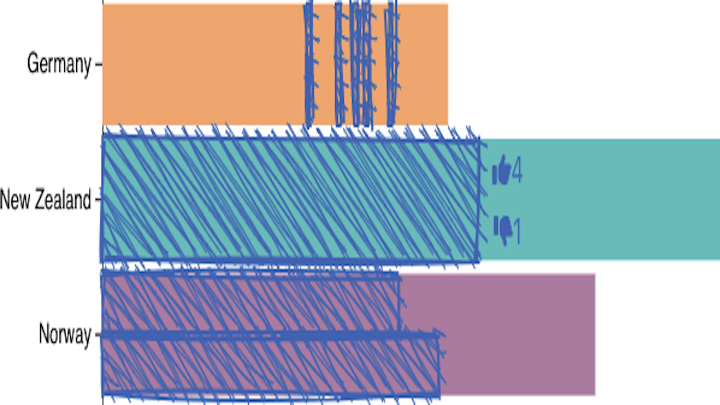
Prerecorded Talk
The live footage of the talk, including the Q&A, can be viewed on the session page, Questioning Data and Data Bias.
Fast forward
Abstract
The trouble with data is that it frequently provides only an imperfect representation of a phenomenon of interest. Experts who are familiar with their datasets will often make implicit, mental corrections when analyzing a dataset, or will be cautious not to be overly confident about their findings if caveats are present. However, personal knowledge about the caveats of a dataset is typically not incorporated in a structured way, which is problematic if others who lack that knowledge interpret the data. In this work, we define such analysts' knowledge about datasets as data hunches. We differentiate data hunches from uncertainty and discuss types of hunches. We then explore ways of recording data hunches, and, based on a prototypical design, develop recommendations for designing visualizations that support data hunches. We conclude by discussing various challenges associated with data hunches, including the potential for harm and challenges for trust and privacy. We envision that data hunches will empower analysts to externalize their knowledge, facilitate collaboration and communication, and support the ability to learn from others' data hunches.