Understanding Missing Links in Bipartite Networks with MissBiN
Jian Zhao, Maoyuan Sun, Francine Chen, Patrick Chiu
External link (DOI)
View presentation:2021-10-28T17:15:00ZGMT-0600Change your timezone on the schedule page
2021-10-28T17:15:00Z
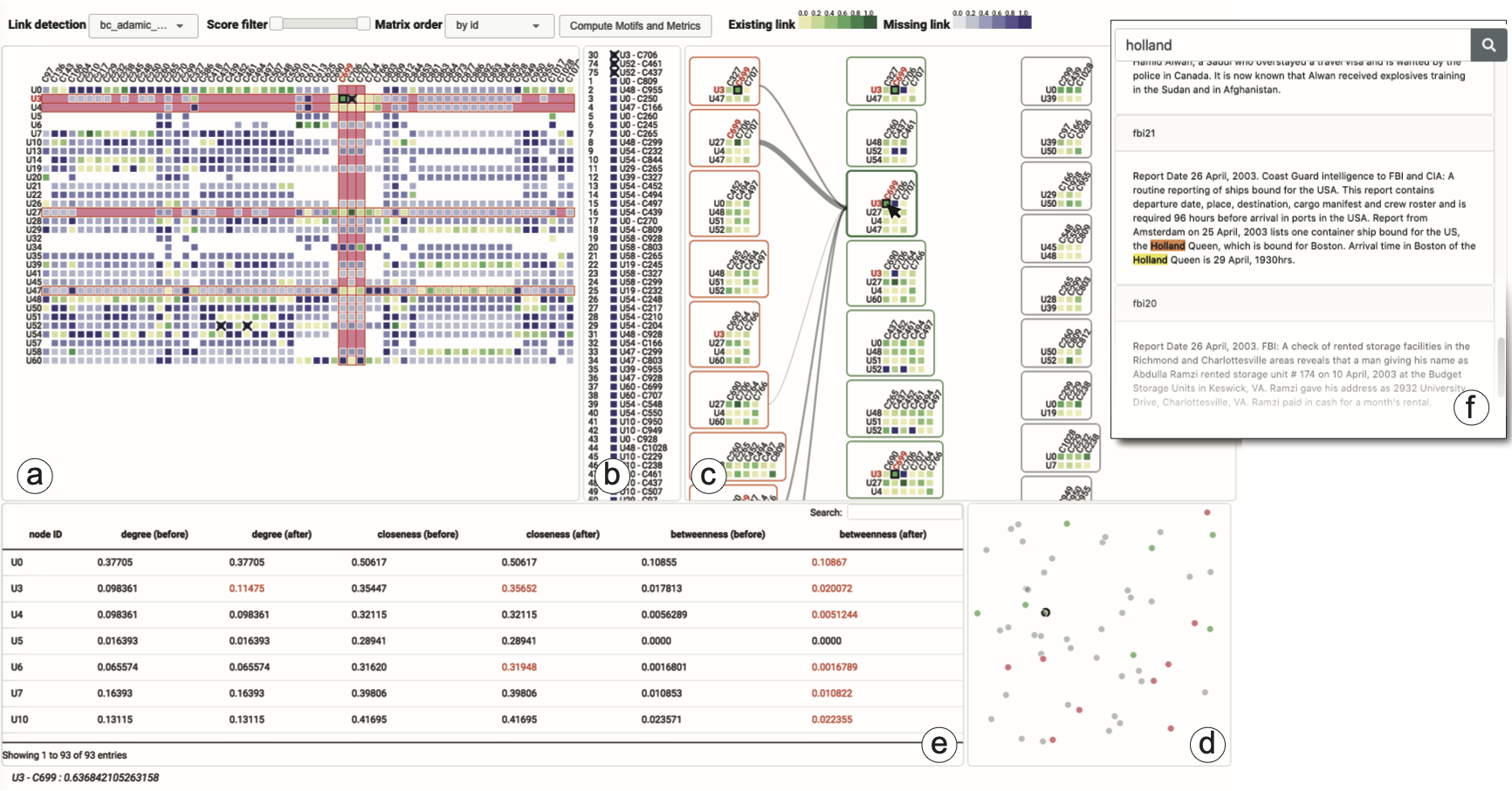
Fast forward
Direct link to video on YouTube: https://youtu.be/qFoHPIJTz0M
Keywords
Missing link prediction, bipartite network, bi-clique, interactive visualization, visual analytics.
Abstract
The analysis of bipartite networks is critical in a variety of application domains, such as exploring entity co-occurrences in intelligence analysis and investigating gene expression in bio-informatics. One important task is missing link prediction, which infers the existence of unseen links based on currently observed ones. In this paper, we propose a visual analysis system, MissBiN, to involve analysts in the loop for making sense of link prediction results. MissBiN equips a novel method for link prediction in a bipartite network by leveraging the information of bi-cliques in the network. It also provides an interactive visualization for understanding the algorithm outputs. The design of MissBiN is based on three high-level analysis questions (what, why, and how) regarding missing links, which are distilled from the literature and expert interviews. We conducted quantitative experiments to assess the performance of the proposed link prediction algorithm, and interviewed two experts from different domains to demonstrate the effectiveness of MissBiN as a whole. We also provide a comprehensive usage scenario to illustrate the usefulness of the tool in an application of intelligence analysis.