Probabilistic Data-Driven Sampling via Multi-Criteria Importance Analysis
Ayan Biswas, Soumya Dutta, Earl Lawrence, John Patchett, Jon Calhoun, James Ahrens
External link (DOI)
View presentation:2021-10-29T15:30:00ZGMT-0600Change your timezone on the schedule page
2021-10-29T15:30:00Z
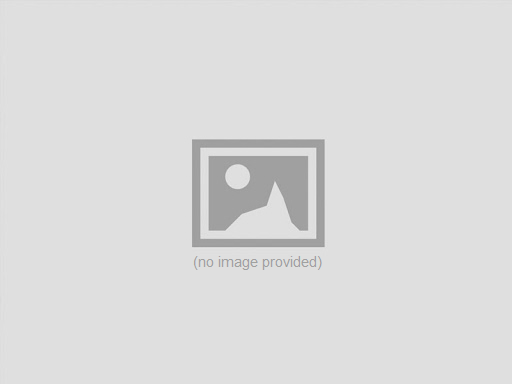
Fast forward
Direct link to video on YouTube: https://youtu.be/NsFQrWT-V14
Keywords
Data visualization, Data models, Computational modeling, Task analysis, Sampling methods, Data analysis, Visualization, Importance sampling, data reduction, error quantification, feature preservation
Abstract
Although supercomputers are becoming increasingly powerful, their components have thus far not scaled proportionately. Compute power is growing enormously and is enabling finely resolved simulations that produce never-before-seen features. However, I/O capabilities lag by orders of magnitude, which means only a fraction of the simulation data can be stored for post hoc analysis. Prespecified plans for saving features and quantities of interest do not work for features that have not been seen before. Data-driven intelligent sampling schemes are needed to detect and save important parts of the simulation while it is running. Here, we propose a novel sampling scheme that reduces the size of the data by orders-of-magnitude while still preserving important regions. The approach we develop selects points with unusual data values and high gradients. We demonstrate that our approach outperforms traditional sampling schemes on a number of tasks.