A Mixed-Initiative Visual Analytics Approach for Qualitative Causal Modeling
Fahd Husain, Pascale Proulx, Meng-Wei Chang, Rosa Romero-Gómez, Holland Marie Vasquez
External link (DOI)
View presentation:2021-10-29T14:10:00ZGMT-0600Change your timezone on the schedule page
2021-10-29T14:10:00Z
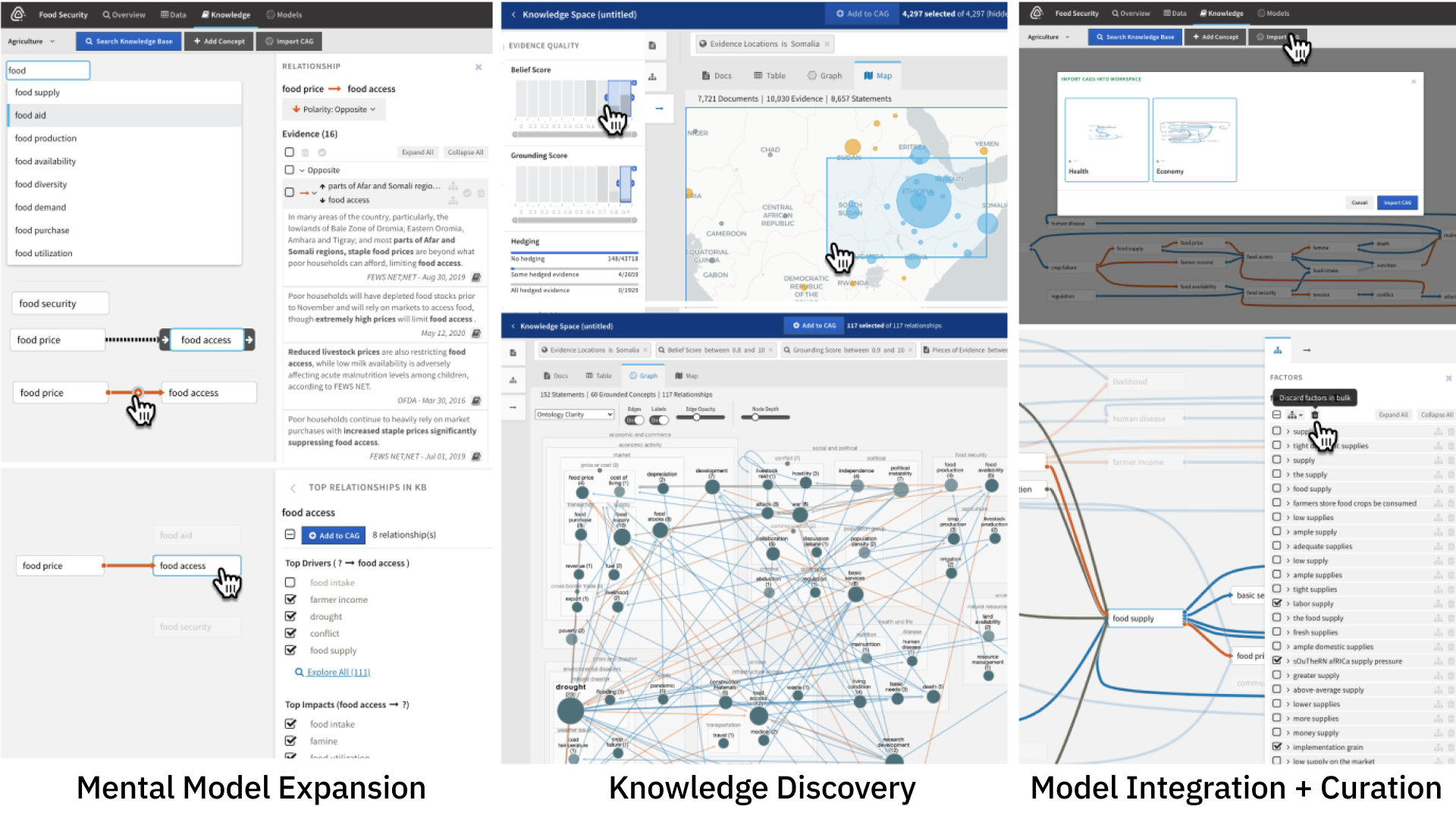
Keywords
Large-Scale Data Techniques, Life Sciences, Health, Medicine, Biology, Bioinformatics, Genomics, Physical & Environmental Sciences, Engineering, Mathematics, Social Science, Education, Humanities, Journalism, Intelligence Analysis, Knowledge Work, Other Topics and Techniques, Mixed Initiative Human-Machine Analysis, Application Motivated Visualization, Graph/Network and Tree Data
Abstract
Modeling complex systems is a time-consuming, difficult and fragmented task, often requiring the analyst to work with disparate data, a variety of models, and expert knowledge across diverse domains. Applying a user-centered design process, we developed Causemos, an integrated visual analytics approach that allows analysts to rapidly assemble qualitative causal models of complex socio-natural systems. Through a mixed-initiative interaction workflow, this approach facilitates the construction, exploration, and curation of qualitative models bringing together data across diverse domains. Referencing a recent user evaluation, we demonstrate Causemos’ ability to interactively enrich user mental models and accelerate qualitative model building.