Compass: Towards Better Causal Analysis of Urban Time Series
Zikun Deng, Di Weng, Xiao Xie, Jie Bao, Yu Zheng, Mingliang Xu, Wei Chen, Yingcai Wu
External link (DOI)
View presentation:2021-10-28T15:45:00ZGMT-0600Change your timezone on the schedule page
2021-10-28T15:45:00Z
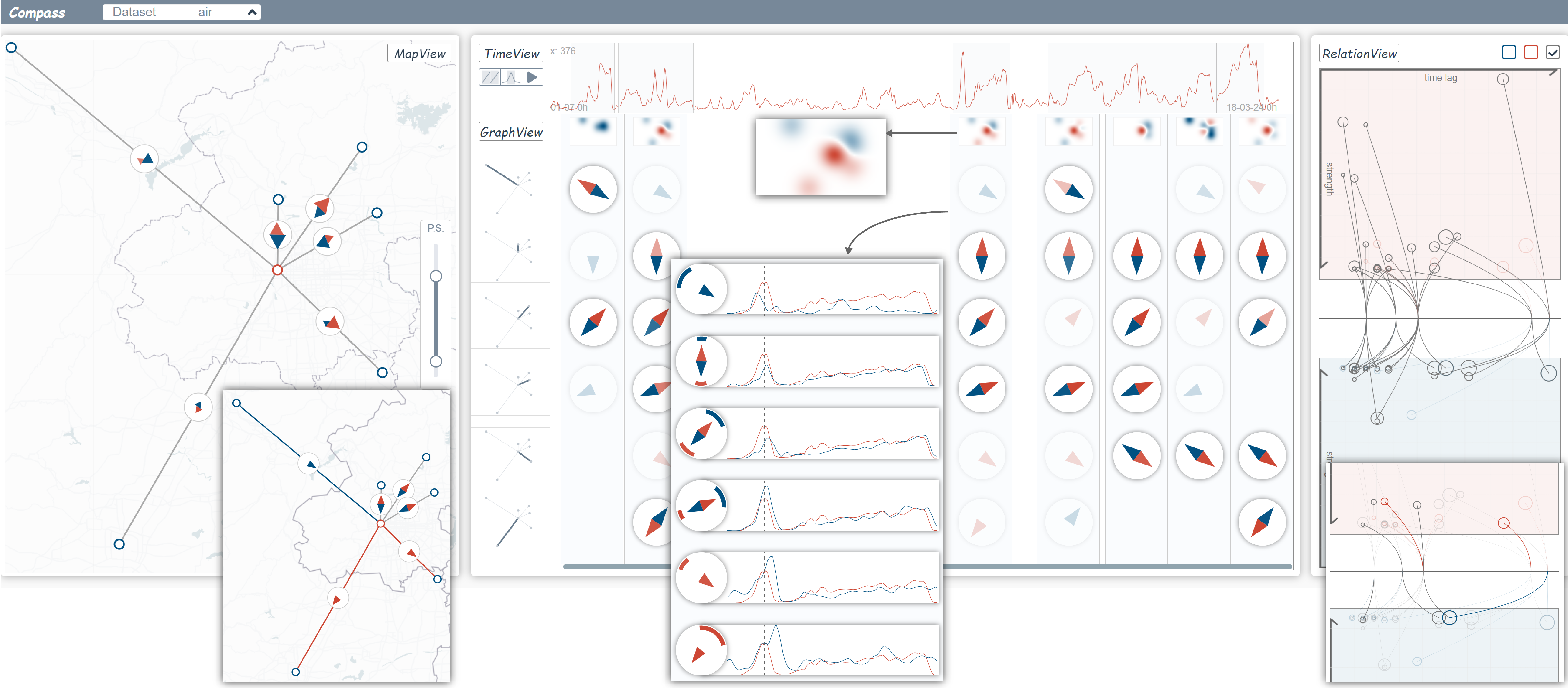
Fast forward
Direct link to video on YouTube: https://youtu.be/XQpSA3iRs7k
Abstract
The spatial time series generated by city sensors allow us to observe urban phenomena like environmental pollution and traffic congestion at an unprecedented scale. However, recovering causal relations from these observations to explain the sources of urban phenomena remains a challenging task because these causal relations tend to be time-varying and demand proper time series partitioning for effective analyses. The prior approaches extract one causal graph given long-time observations, which cannot be directly applied to capturing, interpreting, and validating dynamic urban causality. This paper presents Compass, a novel visual analytics approach for in-depth analyses of the dynamic causality in urban time series. To develop Compass, we identify and address three challenges: detecting urban causality, interpreting dynamic causal relations, and unveiling suspicious causal relations. First, multiple causal graphs over time among urban time series are obtained with a causal detection framework extended from the Granger causality test. Then, a dynamic causal graph visualization is designed to reveal the time-varying causal relations across these causal graphs and facilitate the exploration of the graphs along the time. Finally, a tailored multi-dimensional visualization is developed to support the identification of spurious causal relations, thereby improving the reliability of causal analyses. The effectiveness of Compass is evaluated with two case studies conducted on the real-world urban datasets, including the air pollution and traffic speed datasets, and positive feedback was received from domain experts.