A Visual Analytics Approach to Exploring the Feature and Label Space Based on Semi-structured Electronic Medical Records
He Wang, Yang Ouyang, Quan Li
Room: 104
2023-10-22T03:00:00ZGMT-0600Change your timezone on the schedule page
2023-10-22T03:00:00Z
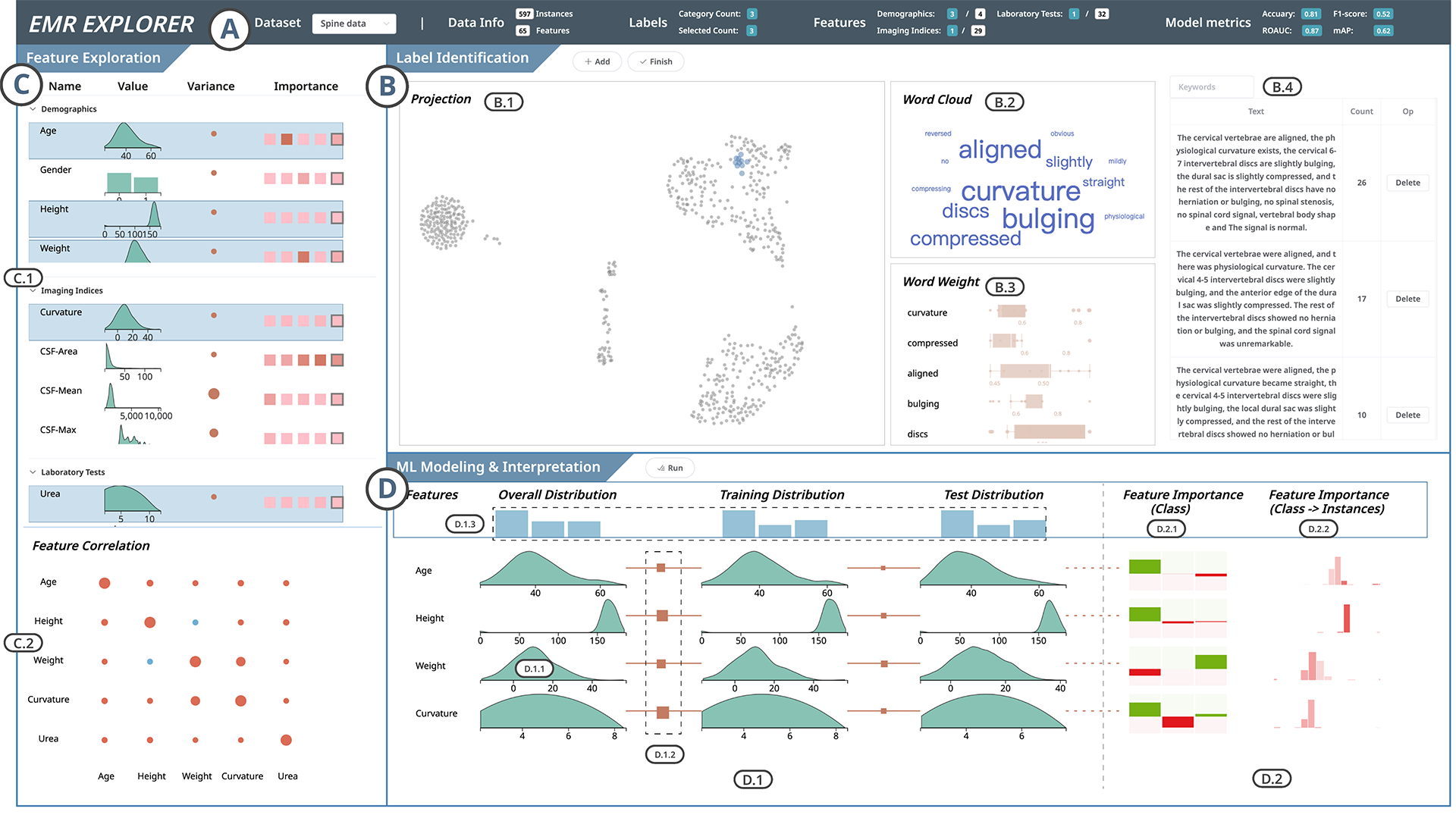
Fast forward
Keywords
Human-centered computing—Visualization—Visualization techniques
Abstract
Electronic health records (EHRs), serving as patient-centered repositories for medical data, offer the opportunity for researchers to uncover concealed patterns using machine learning (ML). However, in real-world medical settings, clinicians often face the task of selecting pertinent feature dimensions from a range of potential medical metrics and then deducing potential labels from vague diagnostic descriptions, prior to the modeling phase. This complexity presents challenges in obtaining reliable training/testing data and conducting thorough analysis. Consequently, these hurdles hinder the practical application of ML for automated modeling and comprehensible interpretation of influencing factors. To tackle these challenges, we introduce a visual analytics approach designed to navigate the feature and label space within EHRs, while also streamlining the modeling process through automated ML algorithms and techniques for improved interpretability.