Deep Hierarchical Super Resolution for Scientific Data
Skylar W. Wurster, Hanqi Guo, Han-Wei Shen, Thomas Peterka, Jiayi Xu
DOI: 10.1109/TVCG.2022.3214420
Room: 106
2023-10-25T23:57:00ZGMT-0600Change your timezone on the schedule page
2023-10-25T23:57:00Z
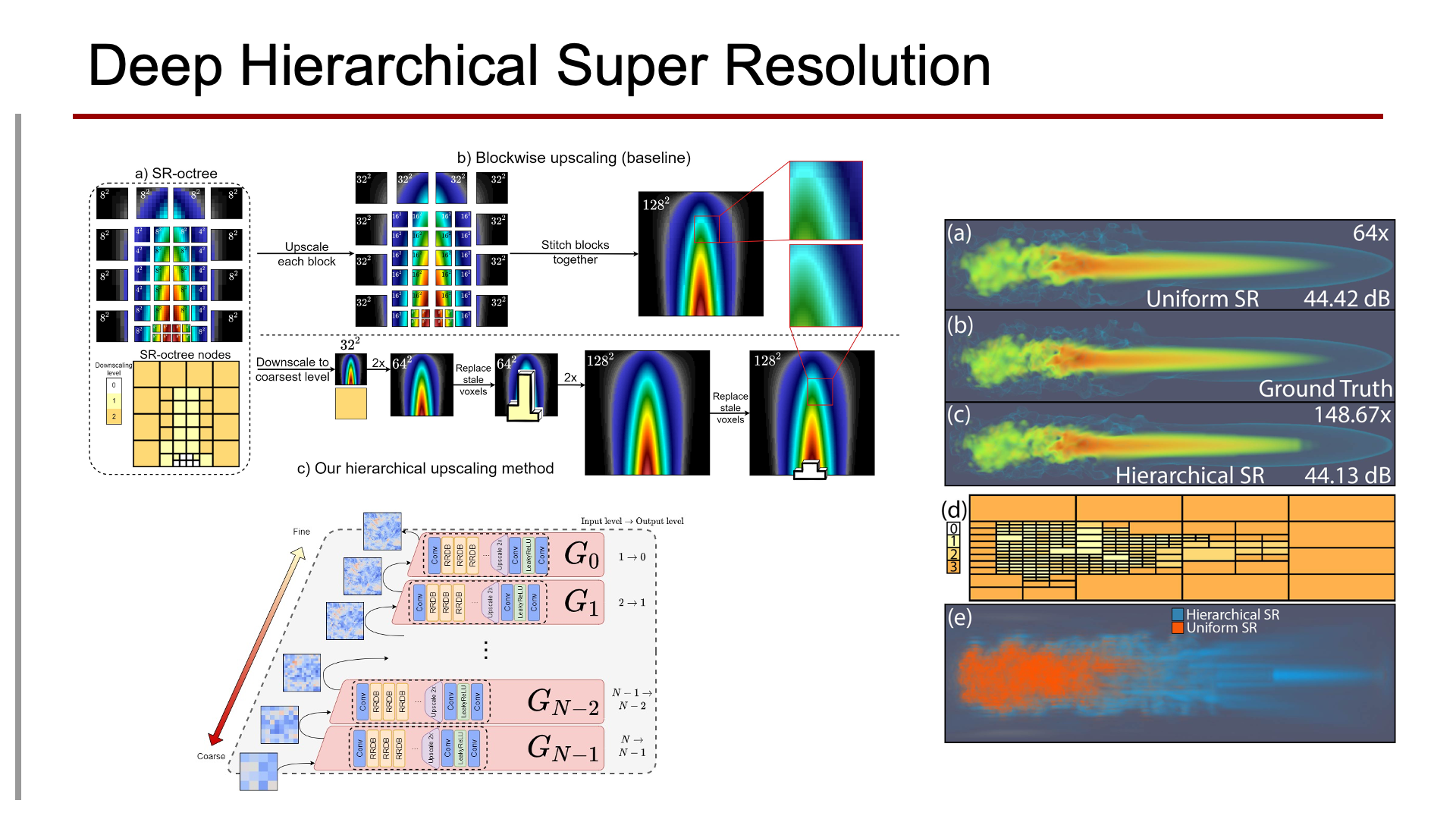
Fast forward
Full Video
Keywords
Deep learning;super-resolution;hierarchical data
Abstract
We present a novel technique for hierarchical super resolution (SR) with neural networks (NNs), which upscales volumetric data represented with an octree data structure to a high-resolution uniform grid with minimal seam artifacts on octree node boundaries. Our method uses existing state-of-the-art SR models and adds flexibility to upscale input data with varying levels of detail across the domain, instead of only uniform grid data that are supported in previous approaches. The key is to use a hierarchy of SR NNs, each trained to perform 2x SR between two levels of detail, with a hierarchical SR algorithm that minimizes seam artifacts by starting from the coarsest level of detail and working up. We show that our hierarchical approach outperforms baseline interpolation and hierarchical upscaling methods, and demonstrate the usefulness of our proposed approach across three use cases including data reduction using hierarchical downsampling+SR instead of uniform downsampling+SR, computation savings for hierarchical finite-time Lyapunov exponent field calculation, and super-resolving low-resolution simulation results for a high-resolution approximation visualization.