IF-City: Intelligible Fair City Planning to Measure, Explain and Mitigate Inequality
Yan Lyu, Hangxin Lu, Min Kyung Lee, Gerhard Schmitt, Brian Y. Lim
DOI: 10.1109/TVCG.2023.3239909
Room: 105
2023-10-26T22:00:00ZGMT-0600Change your timezone on the schedule page
2023-10-26T22:00:00Z
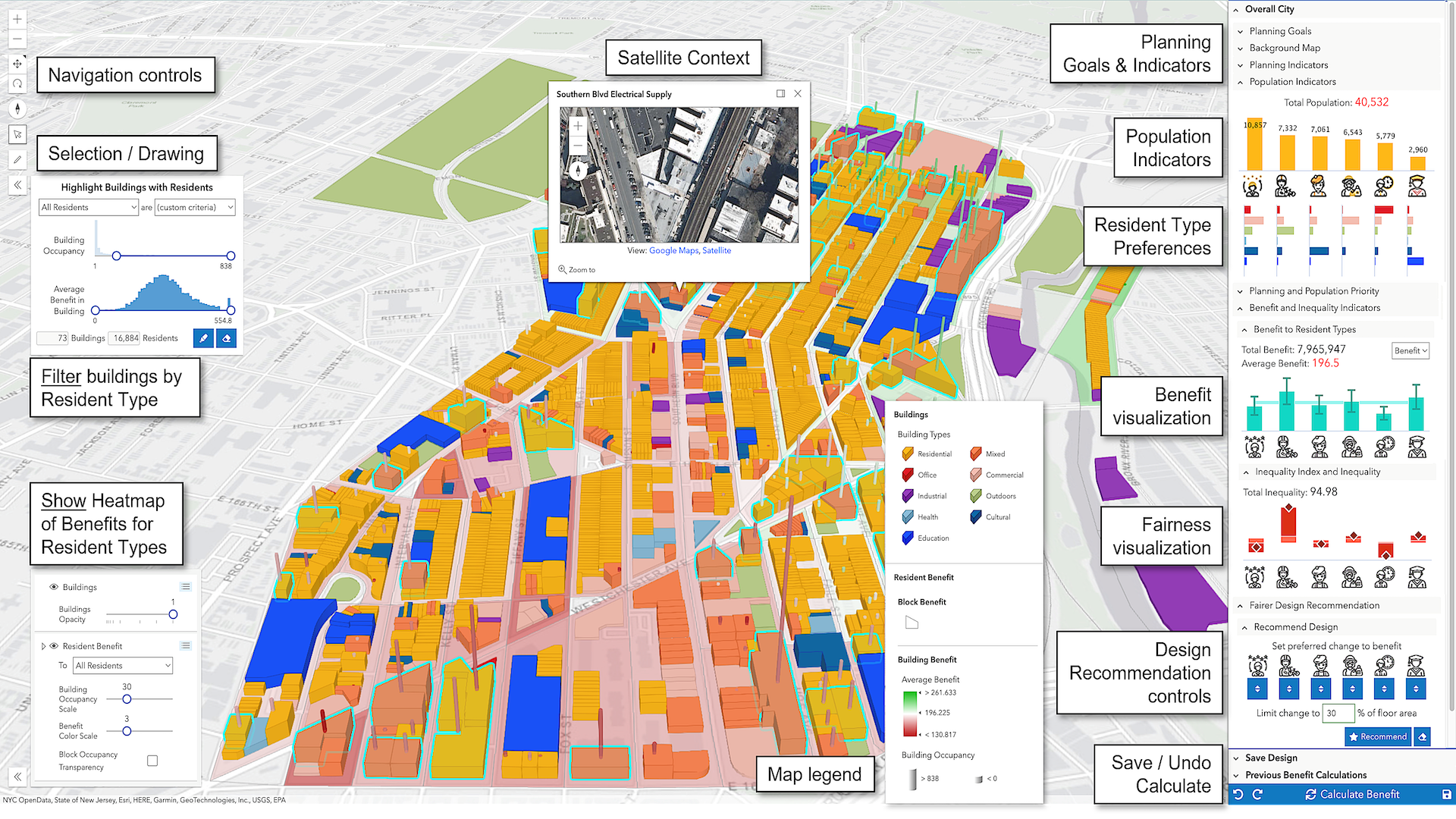
Fast forward
Full Video
Keywords
Explainable artificial intelligence;fairness;intelligibility;resource allocation;urban planning
Abstract
With the increasing pervasiveness of Artificial Intelligence (AI), many visual analytics tools have been proposed to examine fairness, but they mostly focus on data scientist users. Instead, tackling fairness must be inclusive and involve domain experts with specialized tools and workflows. Thus, domain-specific visualizations are needed for algorithmic fairness. Furthermore, while much work on AI fairness has focused on predictive decisions, less has been done for fair allocation and planning, which require human expertise and iterative design to integrate myriad constraints. We propose the Intelligible Fair Allocation (IF-Alloc) Framework that leverages explanations of causal attribution (Why), contrastive (Why Not) and counterfactual reasoning (What If, How To) to aid domain experts to assess and alleviate unfairness in allocation problems. We apply the framework to fair urban planning for designing cities that provide equal access to amenities and benefits for diverse resident types. Specifically, we propose an interactive visual tool, Intelligible Fair City Planner (IF-City), to help urban planners to perceive inequality across groups, identify and attribute sources of inequality, and mitigate inequality with automatic allocation simulations and constraint-satisfying recommendations (IF-Plan). We demonstrate and evaluate the usage and usefulness of IF-City on a real neighborhood in New York City, US, with practicing urban planners from multiple countries, and discuss generalizing our findings, application, and framework to other use cases and applications of fair allocation.