TopoSZ: Preserving Topology in Error-Bounded Lossy Compression
Lin Yan, Xin Liang, Hanqi Guo, Bei Wang Phillips
DOI: 10.1109/TVCG.2023.3326920
Room: 106
2023-10-26T05:45:00ZGMT-0600Change your timezone on the schedule page
2023-10-26T05:45:00Z
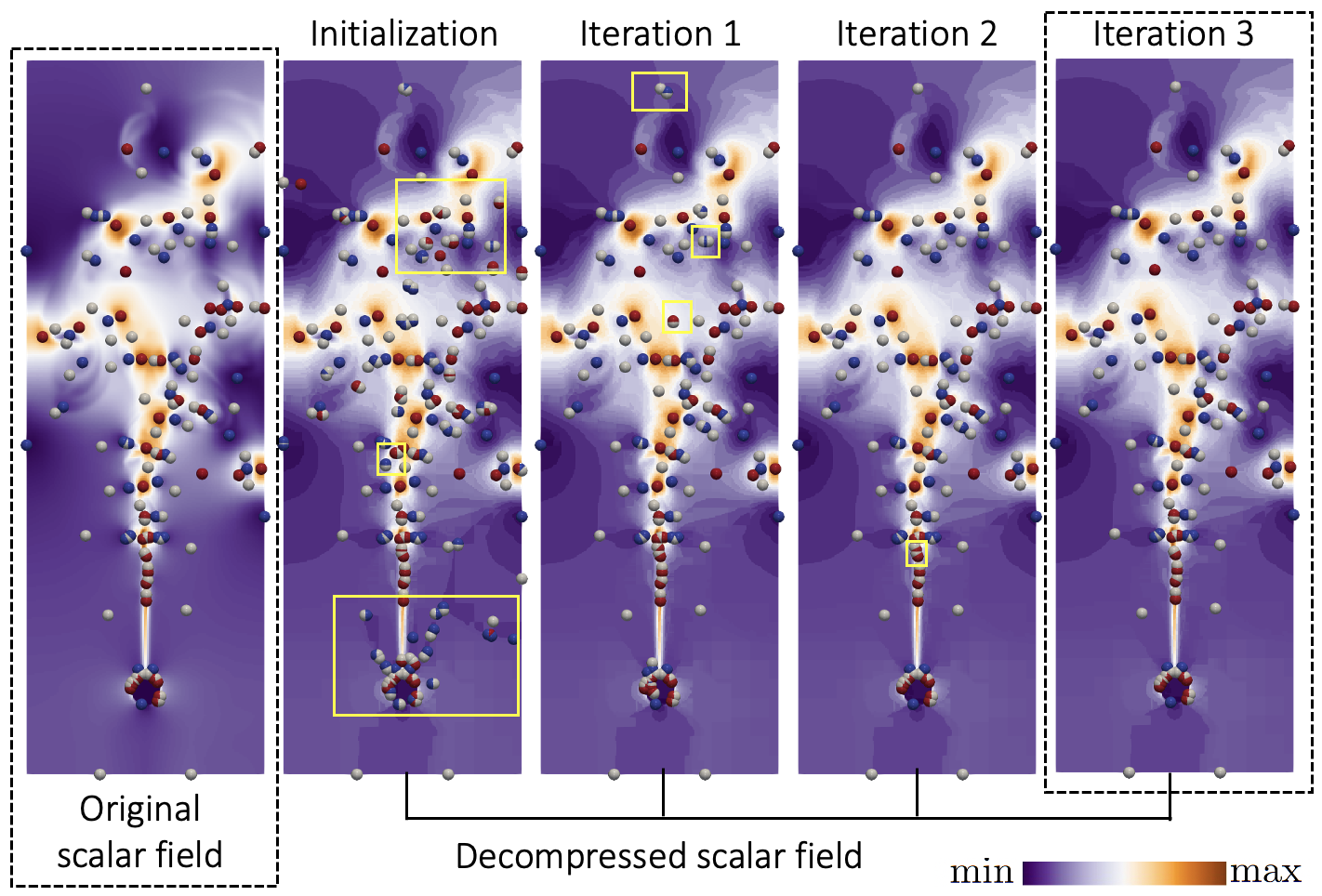
Fast forward
Full Video
Keywords
Lossy compression, contour tree, topology preservation, topological data analysis, topology in visualization
Abstract
Existing error-bounded lossy compression techniques control the pointwise error during compression to guarantee the integrity of the decompressed data. However, they typically do not explicitly preserve the topological features in data. When performing post hoc analysis with decompressed data using topological methods, preserving topology in the compression process to obtain topologically consistent and correct scientific insights is desirable. In this paper, we introduce TopoSZ, an error-bounded lossy compression method that preserves the topological features in 2D and 3D scalar fields. Specifically, we aim to preserve the types and locations of local extrema as well as the level set relations among critical points captured by contour trees in the decompressed data. The main idea is to derive topological constraints from contour-tree-induced segmentation from the data domain, and incorporate such constraints with a customized error-controlled quantization strategy from the SZ compressor (version 1.4). Our method allows users to control the pointwise error and the loss of topological features during the compression process with a global error bound and a persistence threshold.