OW-Adapter: Human-Assisted Open-World Object Detection with a Few Examples
Suphanut Jamonnak, Jiajing Guo, Wenbin He, Liang Gou, Liu Ren
DOI: 10.1109/TVCG.2023.3326577
Room: 109
2023-10-25T05:45:00ZGMT-0600Change your timezone on the schedule page
2023-10-25T05:45:00Z
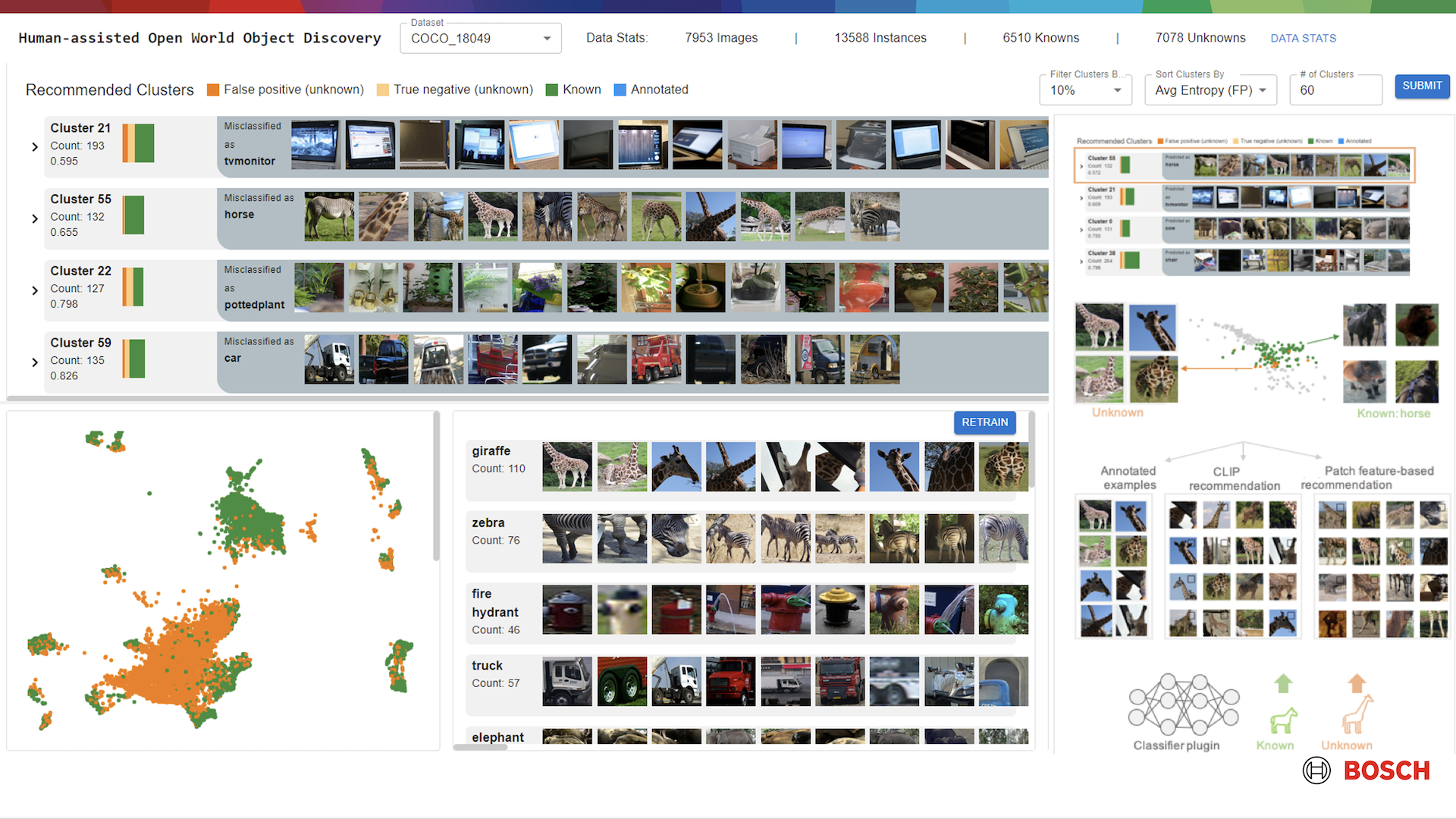
Fast forward
Full Video
Keywords
Open world learning, object detection, continuous learning, human-assisted AI.
Abstract
Open-world object detection (OWOD) is an emerging computer vision problem that involves not only the identification of predefined object classes, like what general object detectors do, but also detects new unknown objects simultaneously. Recently, several end-to-end deep learning models have been proposed to address the OWOD problem. However, these approaches face several challenges: a) significant changes in both network architecture and training procedure are required; b) they are trained from scratch, which can not leverage existing pre-trained general detectors; c) costly annotations for all unknown classes are needed. To overcome these challenges, we present a visual analytic framework called OW-Adapter. It acts as an adaptor to enable pre-trained general object detectors to handle the OWOD problem. Specifically, OW-Adapter is designed to identify, summarize, and annotate unknown examples with minimal human effort. Moreover, we introduce a lightweight classifier to learn newly annotated unknown classes and plug the classifier into pre-trained general detectors to detect unknown objects. We demonstrate the effectiveness of our framework through two case studies of different domains, including common object recognition and autonomous driving. The studies show that a simple yet powerful adaptor can extend the capability of pre-trained general detectors to detect unknown objects and improve the performance on known classes simultaneously.